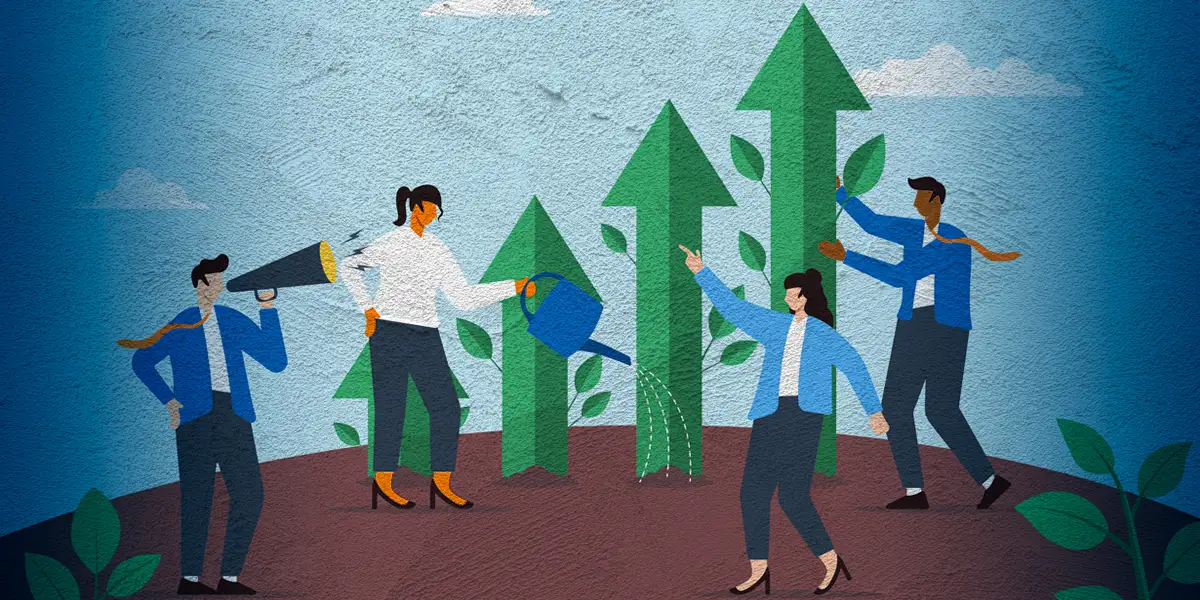
A weak leadership bench can put your company’s future at risk. Learn how to fix it with a succession plan built for long-term success.
How to Build a Succession Plan: A Step-by-Step Guide for HR Leaders
9 min
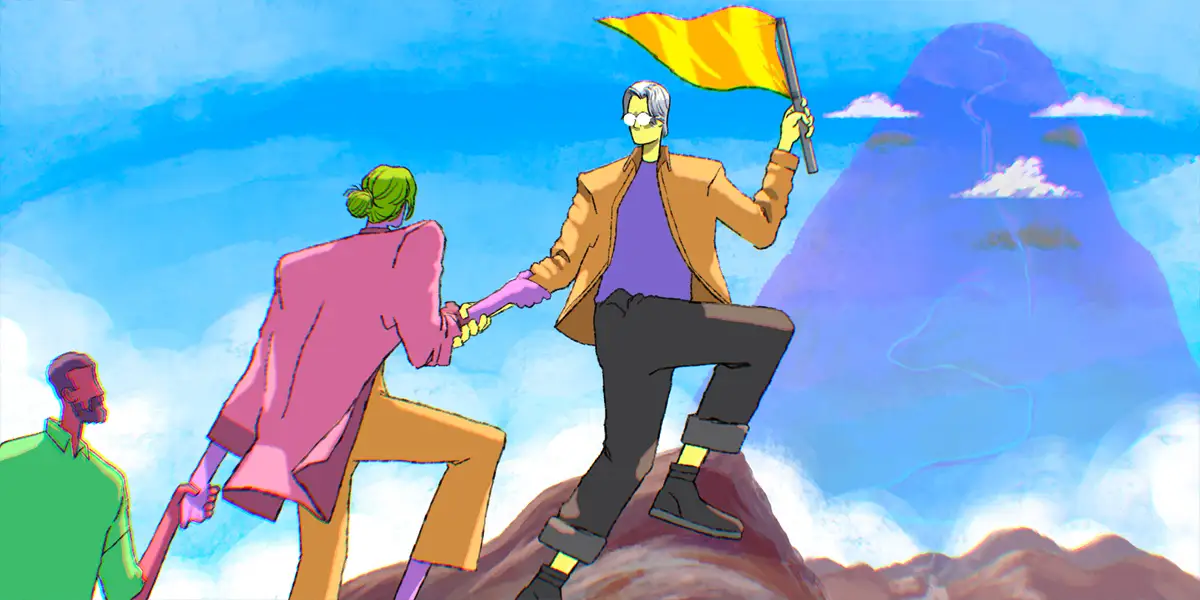
When leaders lose their “why,” engagement slips. Learn why purpose is fading and how to spark meaning for more effective leadership at every level.
The Leadership Purpose Gap: Why Leaders Are Losing Their Sense of Purpose and How L&D Can Fix It
10 min
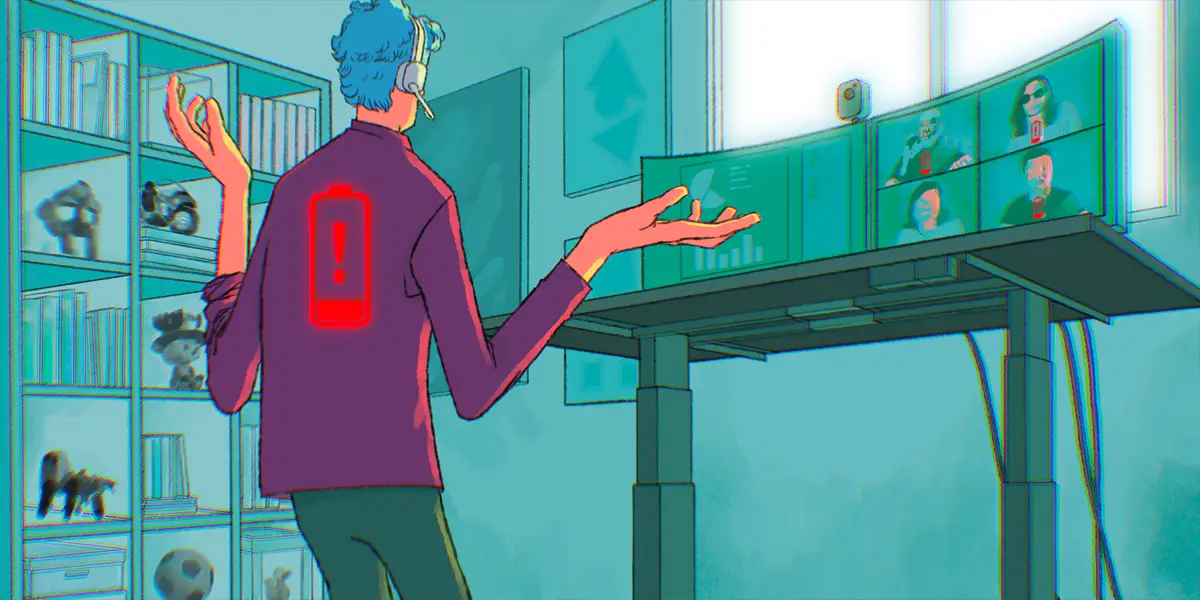
Burnout isn’t just a leadership challenge—it’s a business risk. Discover proven ways to fix workplace issues that drain leaders and build lasting strength.
How to Prevent Burnout in Leaders
11 min
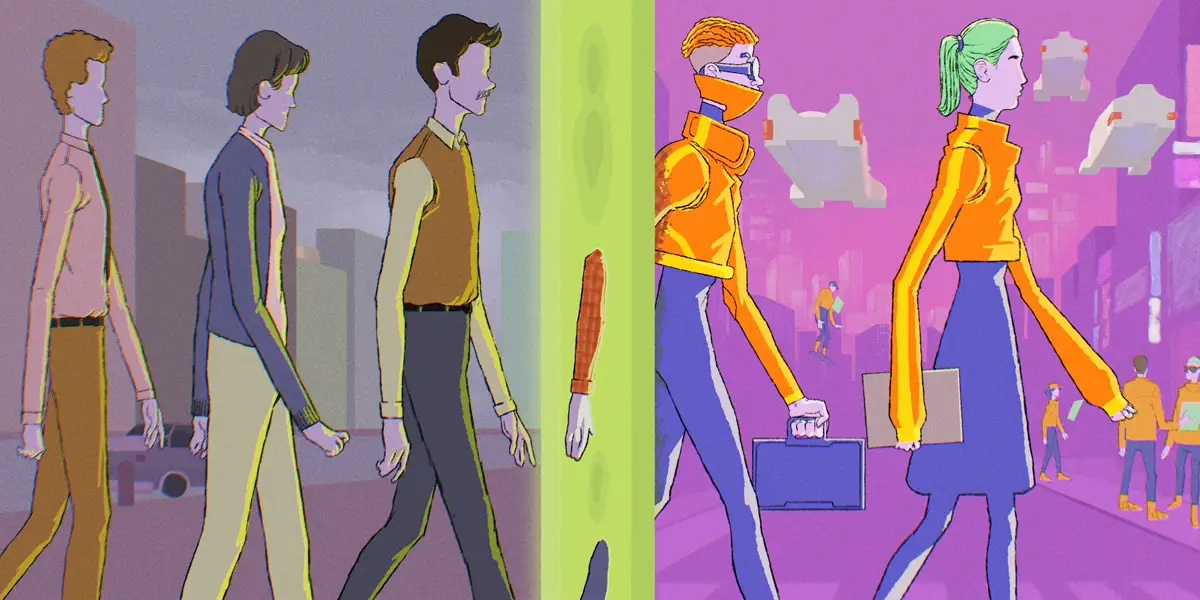
The future of leadership development is written by its past. Learn the challenges of leadership development today and how you should approach it in the times ahead.
The Future of Leadership Development
7 min
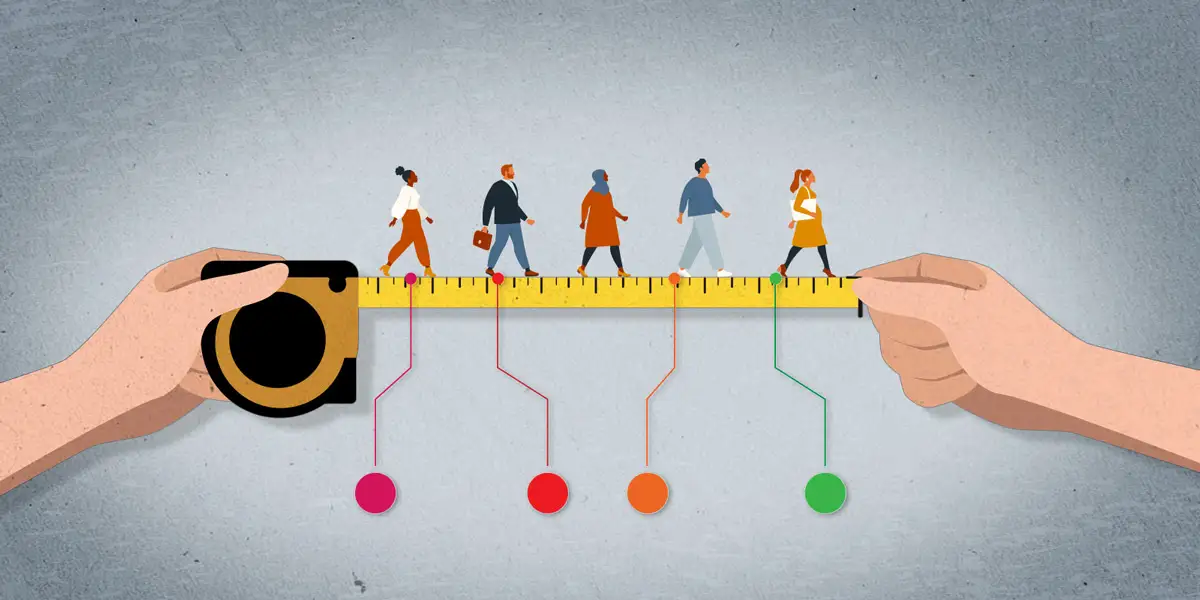
Measuring results of leadership development is a challenge, but it’s not impossible. Learn how to track behavior change, prove impact, and drive business success.
How to Measure Results of Leadership Development
8 min
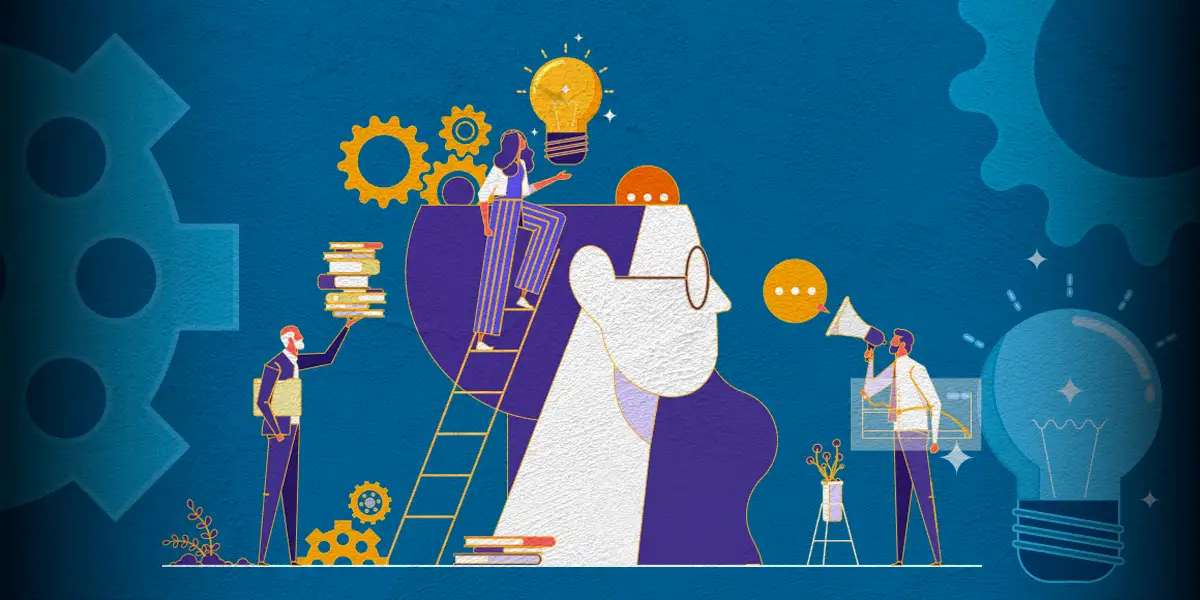
Discover how using assessment for development creates personalized, focused growth for leaders—turning insights into action and building capability and targeted behavior change at every level.
Use Assessment for Development
10 min
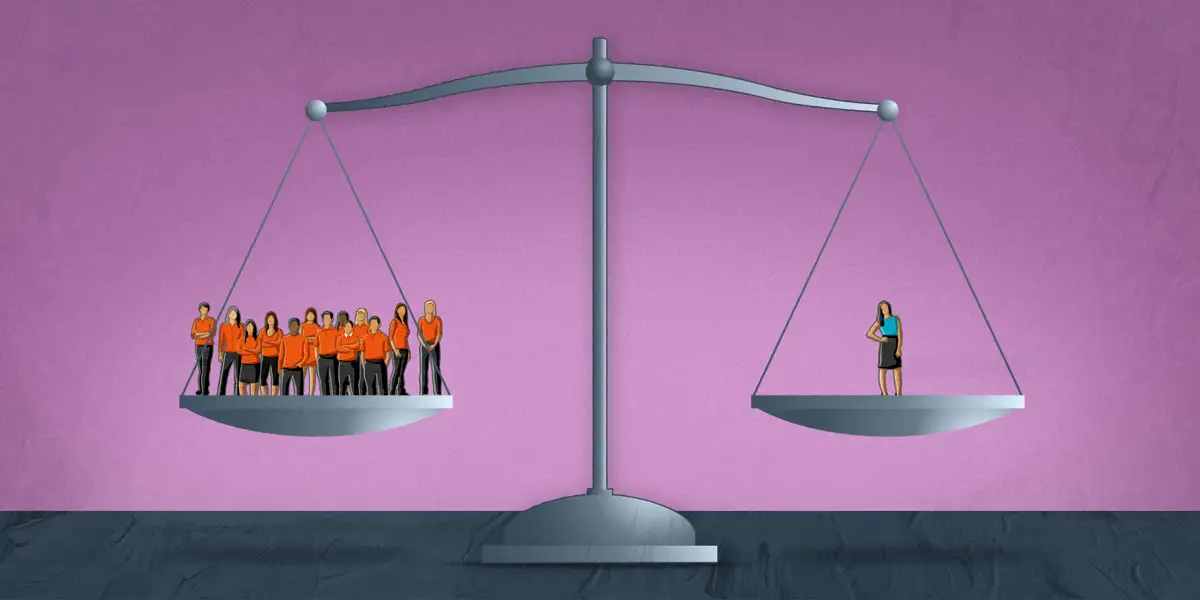
Micro and macro learning work best together—learn how to blend them to create more effective learning journeys.
Micro and Macro Learning: Striking the Right Balance
8 min
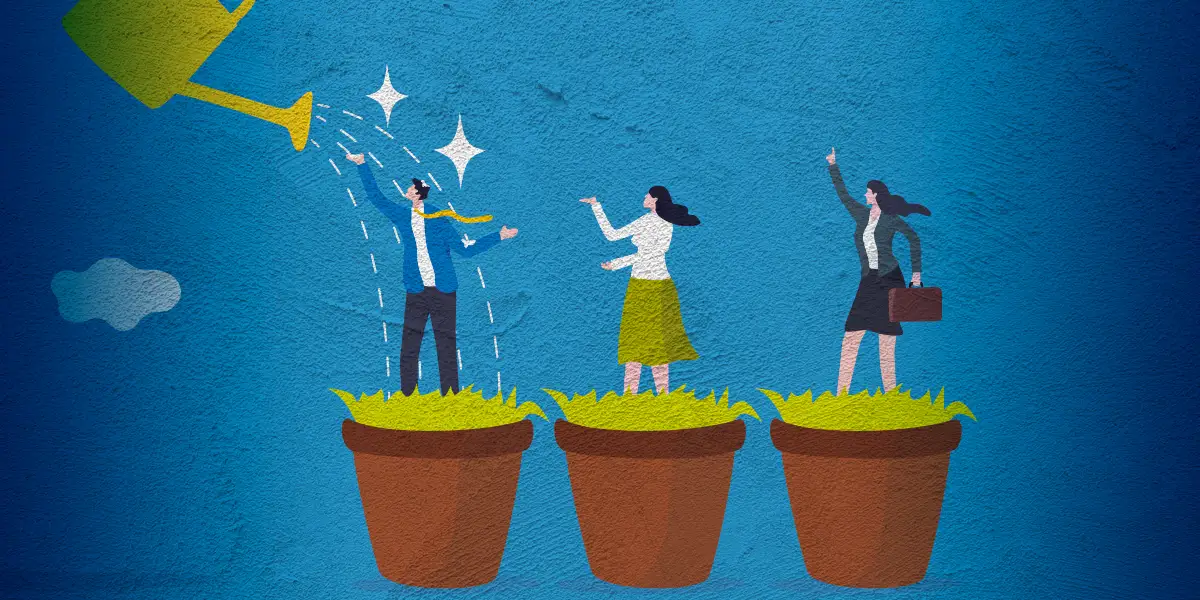
Explore best practices for leadership development that drive real growth. Learn how to design programs that are focused, flexible, and aligned with both leader and business needs.
Best Practices for Leadership Development
7 min